Machine Learning for Real Estate
Machine Learning for Real Estate
Machine Learning for Real Estate
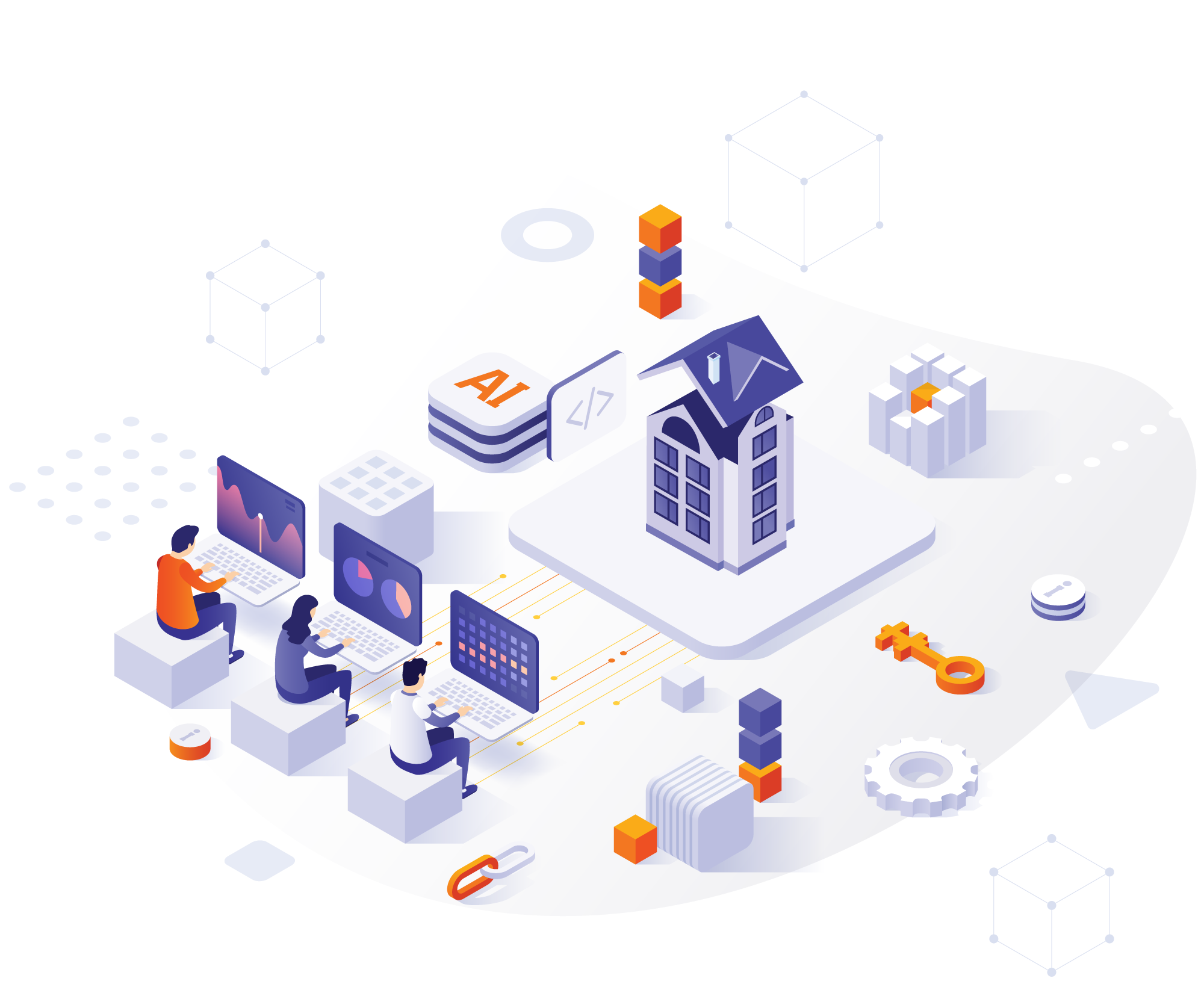
Overview
Real estate is one of the world’s most productive businesses. According to MSCI, the value of business acquisitions worldwide will rise to $9.6 trillion in the coming years Like many other industries, the real estate industry is switching to a “data-driven” world and is creating use cases of artificial intelligence.Further, Real estate has gone virtual as more companies incorporate artificial intelligence into several stages of the buying, selling, and mortgage processes. Morgan Stanley states that real estate is the second least digitized sector globally. However, now just like many other businesses, AI is transforming the landscape of the real estate industry. Additionally, according to the Forbes report, AI is estimated to add a staggering 15.7 trillion US dollars to the world economy by the end of 2030. Artificial intelligence in real estate is a major contributor to the US economy.
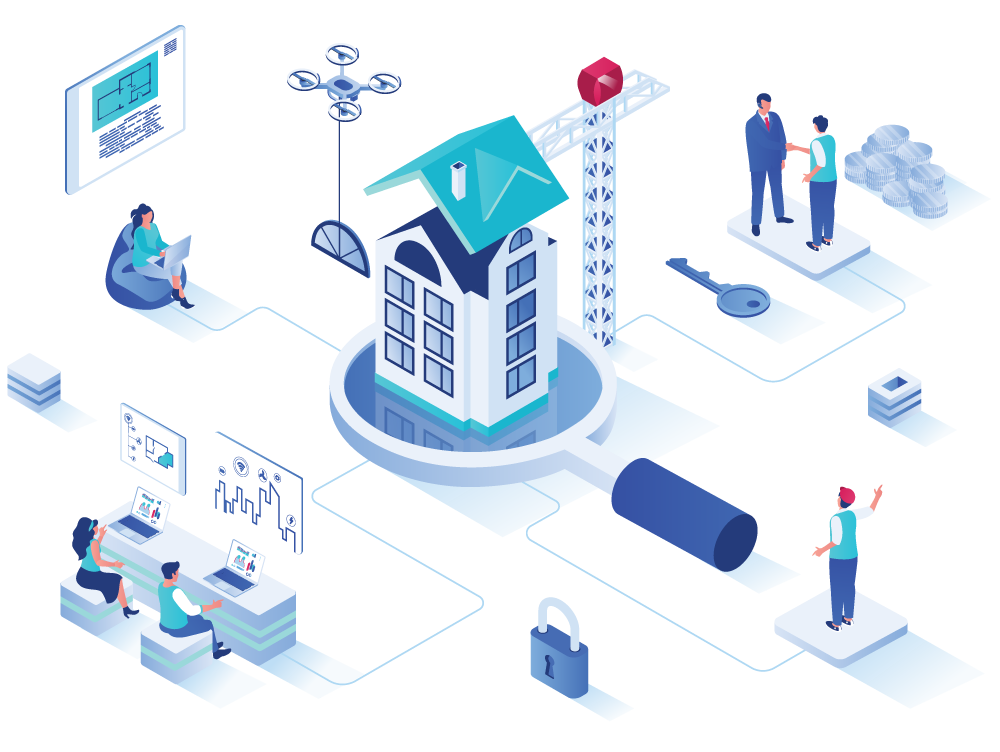
The current state of AI and ML in Real Estate
However, you may feel fine what does it have to do with me? How is it affecting my regular real estate transaction? As a home buyer, real estate agent, home owner how can AI help me make my operations , transactions easy, intuitive and beneficial.
Some of the areas that can be really revolutionized or are being revolutionized using AI and ML are as follows :
01
AI and ML can enable smarter real estate management.
02
AI and ML can help improve the home search experience.
03
AI and ML in real estate can help predict property value.
04
AI and ML can help intensify lead generation.
05
AI Chatbots are also assisting real estate agents in finding the right customers by answering their queries and questions.
06
AI and ML can provide great efficiencies to mortgage lending process
Use Case Under The Scanner
Let us examine some real use cases in more detail. This will throw some light on how AI and ML fare in changing the landscape in the real world.
Smart Home Buying
Real Estate information is generally available to public. However, Real Estate Agents and their offices have to go through the arduous task of sifting through public property records to find title documents, purchase prices, and even mortgage liens. This time-consuming operation has been simplified with the help of computer algorithms that can process millions of records in seconds. Artificial intelligence helps real estate professionals access property values, debt levels, home renovations, and even some of a homeowner’s personal information in the blink of an eye.
Efficient Mortgage Lending
Mortgage lending is one of the biggest parts of the real estate industry, and it is data-intensive by definition. For both banks and customers, this process can be very time-consuming. The customers need to obtain all the documents, and the lenders also need time to process and analyze all that data. Additionally, there must not be a single mistake while processing and analyzing this data because of the astronomical prices of property.
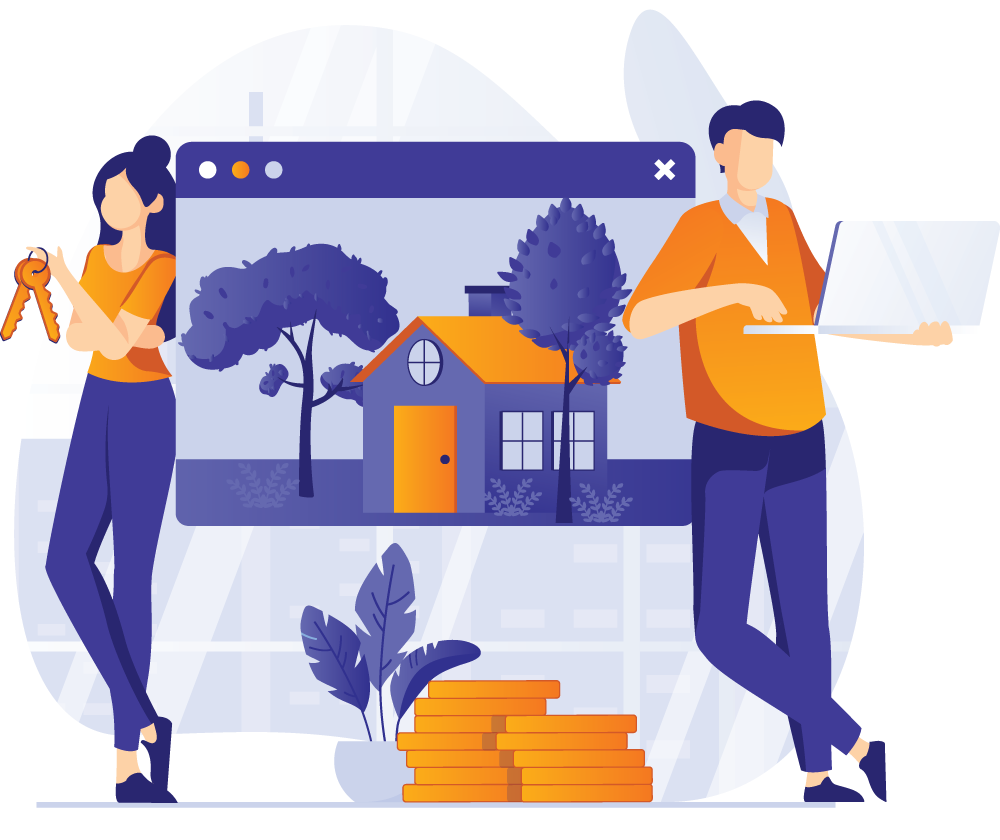
In order to manage this problem, the mortgage lending sector has been using OCR (Optical Character Recognition) technique for decades. However, like many other technologies, it comes with one big limitation. The documents that can only be used for OCR must be in a specific format to come up with accurate data insights.
On the other hand, machine learning techniques and solutions are able to provide more accurate and informative results. Human interference is also negligible, and that’s why more and more lenders are acquiring AI for mortgage lending. According to an estimate, the AI-based solutions provide results that are three times more accurate than earlier methods.
Recommendation Engines based on Customer Preferences
It’s usually very challenging for customers to select the right option according to their unique needs when it comes to renting, selling, or buying real estate. AI technology solves this problem by analyzing customer preferences and providing the most suited available options. The property recommendation engines have already become a reality.
Streamlining Transactions
Many organizations are using such machine learning techniques within their CRMs that generate automatic reports about valuable data insights. It helps real estate agents to learn about their customers instantly and saves their time to manually look for each transaction. Additionally, at the day end, it also helps brokers to assess their performance and customer satisfaction accurately.

How can AI help Real Estate Investors?
Many organizations are using such machine learning techniques within their CRMs that generate automatic reports about valuable data insights. It helps real estate agents to learn about their customers instantly and saves their time to manually look for each transaction. Additionally, at the day end, it also helps brokers to assess their performance and customer satisfaction accurately.
Artificial intelligence helps with decision-making.
Artificial intelligence provides software solutions with the capability of predictive analytics. It offers more insights into the current and future value of any property. This information can be very useful for investors.
Artificial intelligence can help investors gain a competitive edge.
The predictive analytics feature of AI not only helps investors to save money, but it can also gain them a competitive edge. In simple words, if an AI solution can help investors to find out the right value of any property, then it can also indirectly help them to decide what they should offer. If an investor comes up with the right and educated offer, they will automatically be able to gain a competitive edge.
Artificial intelligence automates the investing procedure.
The real estate investment and property management systems powered with AI work on the same principles as the artificially intelligent CRM (Customer Relationship Management) does. As a CRM helps real estate agents to minimize the number of errors and streamline the transaction process, it can also help investors to automate the investing procedure.
ML Real Estate Valuation – An AI and ML Affair
Machine-Learning (ML) holds great promise for real estate valuation. But relevant, high quality and timely real estate data remain an expensive input. Without the data deluge already available for other assets, a balanced mix of modeling and data remains the most likely avenue for property valuation in the next years. Here we offer a birds eye view of how valuations are carried for property selling and property renting from a machine learning modelling perspective.
Some of the insights are as follows :
01
Real estate prices are notorious for their cyclical behaviour across long periods of time, in both developed and developing markets.
02
Cycle duration and amplitude vary across time and have different features across countries.
03
Changes in structural characteristics of both real estate markets (going from local construction and zoning legislation) and credit markets (deregulation and changes in regulatory risk limits) influence heavily to alter the statistical properties of property cycles.
04
Interaction between GDP, credit and real estate represents a valuable source of information regarding the future direction of the property cycle.
So the questions that crop up in a modelling process based on the facts mentioned above are as follows :
01
How and when do these changes show up in the aggregate real estate price data?
02
Do these changes impact forecasting?
03
How should the real estate valuation exercise reflect these changes?
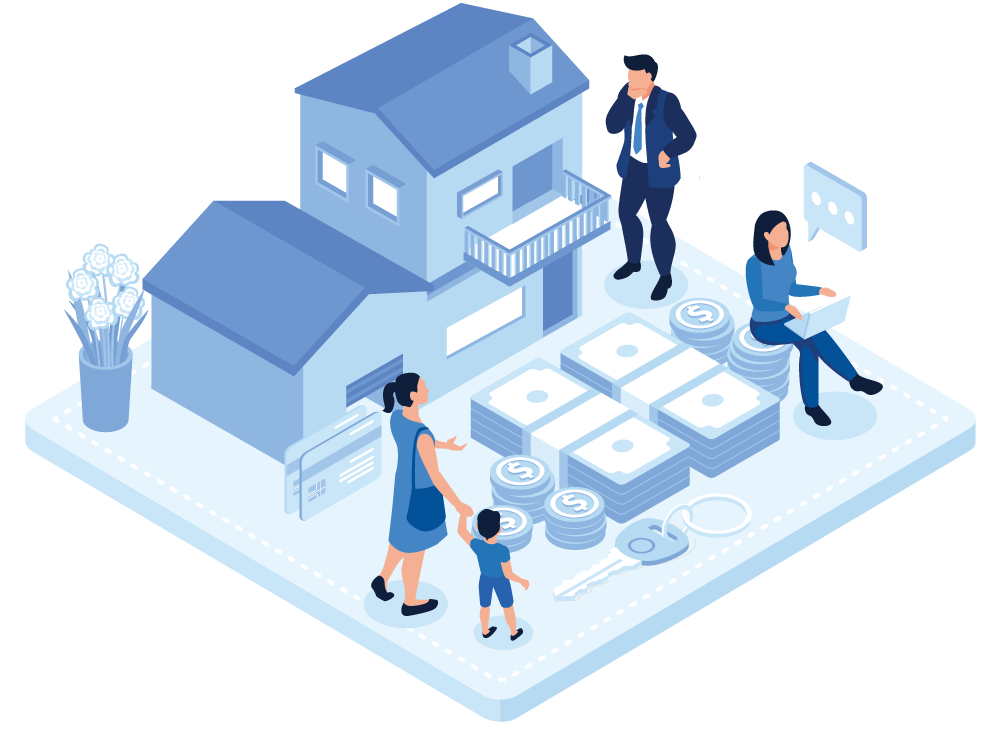
ML Real Estate Valuation – An AI and ML Affair
Machine-Learning (ML) holds great promise for real estate valuation. But relevant, high quality and timely real estate data remain an expensive input. Without the data deluge already available for other assets, a balanced mix of modeling and data remains the most likely avenue for property valuation in the next years. Here we offer a birds eye view of how valuations are carried for property selling and property renting from a machine learning modelling perspective.
Some of the insights are as follows :
01
Real estate prices are notorious for their cyclical behaviour across long periods of time, in both developed and developing markets.
02
Cycle duration and amplitude vary across time and have different features across countries.
03
Changes in structural characteristics of both real estate markets (going from local construction and zoning legislation) and credit markets (deregulation and changes in regulatory risk limits) influence heavily to alter the statistical properties of property cycles.
04
Interaction between GDP, credit and real estate represents a valuable source of information regarding the future direction of the property cycle.
So the questions that crop up in a modelling process based on the facts mentioned above are as follows :
01
How and when do these changes show up in the aggregate real estate price data?
02
Do these changes impact forecasting?
03
How should the real estate valuation exercise reflect these changes?
As an example if you take a 10 yrs period, then the following steps can be performed to prepare a model :
01
Compute the 10-year moving average of growth rates of residential real estate prices, GDP/capita and credit to non-financial corporations.
02
ML may be employed here to search for the optimal window size minimizing forecasting error, as estimated gaps are used in the forecasting model.
03
The 10-year average may be considered as a basic estimate of the underlying trend. This is the low-frequency component of the data.
04
The cyclical component is then obtained by subtracting the trend value from the observed value at all points in time.
05
Additionally, a view of the joint dynamic across countries may be obtained by estimating a Loess curve across the available cross-section of 10-year averages
06
In a cross-country setting, ML offers a natural choice for the selection of optimal weights used to compute the global average.
07
Further, selecting a time-varying matrix of coefficients improves results for a forecasting exercise.
08
Naturally, for each market, the estimation may be further refined using with country-specific individual transaction data.
This is a straightforward application aimed at revealing the presence of global factors across real estate, GDP and credit growth.
Conclusion
The power of AI to help real estate companies in many important ways is disruptive. Decision-makers will focus on additional case studies to improve the real estate industry, such as other AI divisions. However, when firms quickly understand how to apply data science to their organizations, both customers and owners will be more productive.
Embracing AI in real estate is an ongoing process rather than just starting; businesses should set their long-term goals and use AI tools to achieve their destination in less time and with greater clarity.
AI will not replace human beings, but it does play an important role. People buy not property; they buy their personal and emotional decisions to feel comfort in their comfortable home. In the future, therefore, Emotional AI should be developed to study human emotions in-depth and see the emotional implications of home elections.
Have an Inquiry?

Mithun S
Mithun is an avid technology geek at heart and is deeply interested in AI, Metaverse and other emerging technologies. He heads the R&D Practice at AARCHIK Solutions